In the ever-evolving world of machine learning, a new model has emerged that is set to redefine the boundaries of image and audio classification. This model, known as ByteFormer, is the subject of a recent research paper titled “ByteFormer: Byte-Level Vision and Beyond“. What sets ByteFormer apart is its unique ability to consume only bytes, without explicitly modeling the input modality. This transformative approach has far-reaching implications for the field of machine learning and beyond.
The Technical Breakthrough
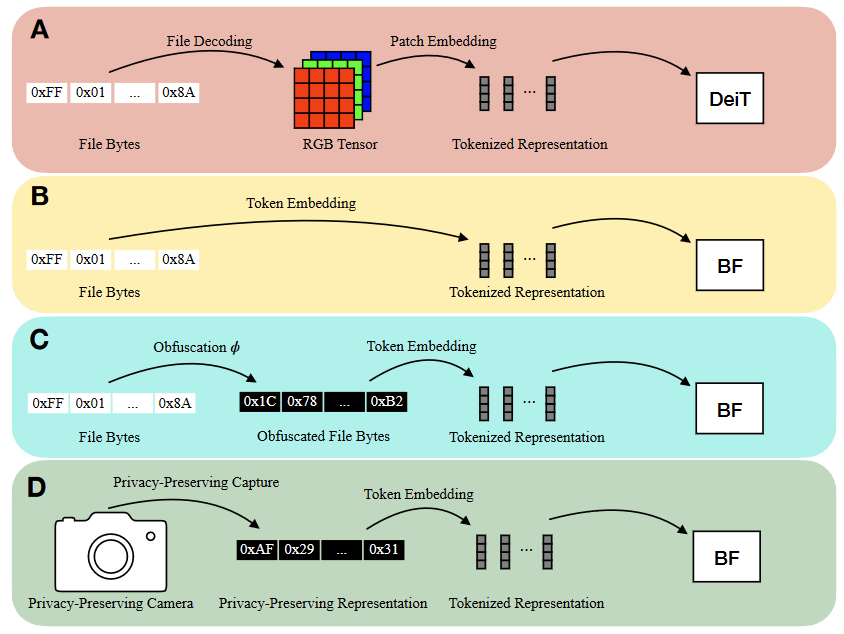
Traditionally, machine learning models have been designed to process specific types of data, such as images or audio. These models require specific input modalities and often need hyperparameter tuning or architecture modifications to perform optimally. ByteFormer, however, breaks away from this norm.
ByteFormer operates at the byte level, meaning it processes raw bytes of data without any prior knowledge about the type of data it’s processing. This is a significant departure from traditional models, which usually require data to be in a specific format or structure.
The research paper demonstrates that ByteFormer achieves strong performance on image and audio classification tasks without any hyperparameter tuning or architecture modifications. This is a remarkable achievement, as it suggests that the model is versatile and adaptable, capable of handling different types of data without needing specific adjustments.
The Power of Byte-Level Processing
The ability to process data at the byte level opens up a world of possibilities. For instance, ByteFormer can be used in conjunction with image obfuscation techniques with little or no loss in accuracy. Image obfuscation is a method used to protect privacy by altering images so that they cannot be recognized by conventional image recognition systems. By being able to work effectively with obfuscated images, ByteFormer can play a crucial role in privacy-preserving applications.
Privacy-Preserving Cameras
One of the most exciting applications of ByteFormer is its potential use in privacy-preserving cameras. These cameras can perform inference without forming a full image at capture time. This means that they can analyze and understand the scene they are capturing without actually creating a detailed image. This has significant implications for privacy, as it means that sensitive information can be protected while still allowing the camera to perform its function.
The Future of Machine Learning
The development of ByteFormer represents a significant step forward in the field of machine learning. Its ability to process data at the byte level, without requiring specific input modalities, makes it a versatile and powerful tool. Furthermore, its potential applications in privacy-preserving technologies make it a highly relevant and timely innovation.
As we continue to generate and process vast amounts of data, models like ByteFormer will become increasingly important. They offer a way to handle diverse types of data efficiently and effectively, while also addressing critical issues around privacy and data protection.
In conclusion, ByteFormer is not just a novel idea; it’s a transformative approach to machine learning that has the potential to reshape the field. As we continue to explore its capabilities and applications, we can expect to see even more innovative and exciting developments in the future.